Amy Hawksworth
Senior Manager of Downstream Process Sciences, Pharmaron
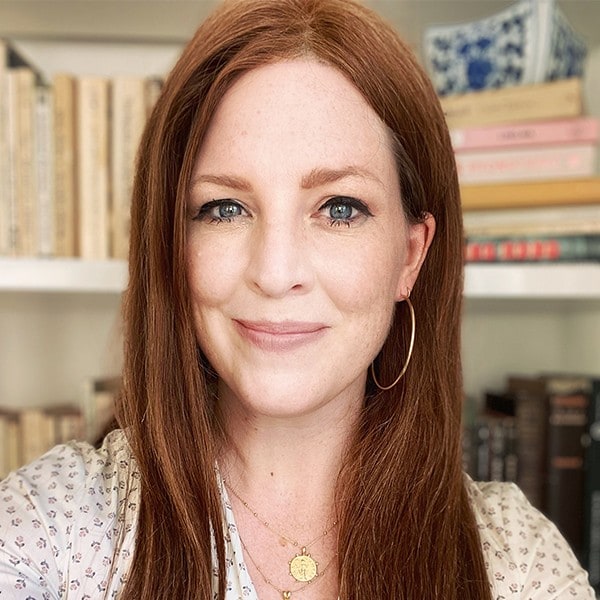
Meg Hermes
Customer Alliance Manager, JMP
Pharmaron is a leading, fully-integrated pharmaceutical R&D services provider with global operations. It has a well-established team of over 20,000 employees working in 21 different sites worldwide.
Amy Hawksworth is Senior Manager in Downstream Process Sciences at Pharmaron’s Gene Therapy CDMO in Liverpool. She has worked in the pharmaceutical industry for 15 years – 11 of those in biologics with roles in early phase drug discovery, both upstream and downstream process development, technical transfer and even a stint working on analytical in vitro assays in QC.
“I am responsible for managing both internal innovation and external client projects to help deliver downstream process development solutions,” Amy explains. “In addition, I manage a number of scientists and enjoy the opportunity to develop their careers in a dynamic and fast-paced scientific organization.”
At present, Amy and her team are working on what is still a relatively new area of medicine – gene therapy. They use adeno-associated virus (AAV) as a vector to deliver a functioning gene to a targeted tissue in the body, thereby producing a missing or non-functioning protein. “This exciting world of advanced therapies allows us to deliver life-changing products to patients to drastically enhance or save lives,” she adds.
Amy sat down with JMP Customer Alliance Manager Meg Hermes to discuss analytics enablement at Pharmaron in Liverpool and share how the company’s adoption of more advanced, data-driven approaches have accelerated drug discovery, development and commercialization.
Meg: What sets Pharmaron apart from other CDMOs?
Amy: At the Liverpool UK site, we primarily work on viral vectors for gene therapy development and clinical manufacture, delivered through our MHRA IMP-licensed cGMP facility. We have a strong team of around 200 people with a high level of expertise in key areas across the business, focused on fulfilling our clients’ project needs through scientific excellence and collaboration.
We are particularly proud of our world-class analytical capabilities delivered through state-of-the-art equipment and expertise in combination with our highly robust platform processes and high-throughput development.
You asked what sets Pharmaron apart from other CDMOs and I think it is our end-to-end integrated services and the quality of our platform process and analytics. Within 6 weeks we can identify if there is a need for process optimization of a client’s product. This offering is very powerful as it really shortens the time in development. Plus, our process has been designed with the end in mind to scale from the lab to clinical manufacturing. So, these two things combined means we can minimize the time to clinical supply and deliver innovative medicines to our patients, faster.
Meg: What is the philosophy of Pharmaron technical leadership when it comes to analytics enablement?
Amy: At Pharmaron Liverpool, we try to let the data speak for itself and ensure we are doing ‘good science’ by using the data to drive our decision making. That means we need to maximize our understanding of the data we obtain.
Meg: So how might that play out in a typical data workflow?
Amy: Data may be generated from a varied range of sources, such as upstream and downstream processing, and from different scales and stages of a product lifecycle. Equally from our plethora of analytical methods where we obtain data from several orthogonal assays which are reported out in different ways.
To handle this, we employ a tiered approach to analytical testing which helps generate more concise data from the start. Firstly, we screen all samples generated from process development HTP studies using fast, HTP assays such as ELISA or SEC for titre to gain a quick understanding of the landscape of our study. Based on these results, we would then select a subset of samples to take forward to intermediate testing to generate more accurate data and gain information from a wider number of assays. And finally, we would execute extended testing to characterize a small subset of samples more thoroughly, through potentially more costly assays but with higher accuracy.
This approach leads to a better use of resources, faster time to results, and earlier decision making. Once we have obtained the data from this tiered testing approach, we utilize a strategy of thorough data collation, data preparation, data analysis and data presentation. We use a pre-defined master data spreadsheet to ensure data is collated with the right structure and formatting in mind. This allows us to collate, combine and transform the data for accurate data analysis in a short space of time.
Such a streamlined internal approach permits us to quickly obtain meaningful insights from our data and provide opportunities for further optimization of our process.
Meg: What are some of the key data challenges your area of the business is facing right now?
Amy: One of the key challenges for our business and the sector as a whole is the sheer amount of data generated from our studies. We are working on complex biological systems with a multitude of naturally varying factors which could be impacting results at any one time.
As a result, simple data modeling and OFAT (one factor at a time) methods are not appropriate. Instead, we move to testing multiple factors simultaneously under one experiment; which can be complex to design. In addition, we are using high-throughput systems to conduct these experiments – and have equivalent high-throughput analytics to examine the resulting samples. Ultimately, the result is a huge data set from just one experiment.
Dealing with so much data can be daunting and simply adding the data to a spreadsheet – and manually looking through it for trends, outcomes, and discrepancies – does not suffice.
Meg: What are you doing to respond to the challenge of massive data sets?
Amy: The team at Pharmaron Liverpool now regularly use JMP® throughout the experimental life cycle to help us overcome this challenge. JMP has helped to define study goals and optimize our resource inputs using design of experiments (DOE) approaches.
JMP has allowed Pharmaron to gather data in a standardized way and to analyze that data quickly to highlight areas for optimization and improvement. It has also enabled our scientists to conduct statistical analysis, find trends and outliers, and present the data back in a format ready to share in presentations and write-ups. This drives the quality and quantity of the products we produce.
Meg: Can you share an example of how JMP has helped improve scientific outcomes or accelerate the timeline for patients to get access to Pharmaron therapies?
Amy: At Pharmaron Liverpool, we have a platform process that allows us to make drug substance AAVs for multiple products. Depending on the product, we can make adjustments and improvements to the process using our toolbox-based approach to fine tune a number of parameters for targeted optimization.
For these process improvements, we routinely use JMP to help conduct a DOE where we examine multiple factors concurrently. One simple example of this is when optimizing our capture chromatography step in our downstream process by assessing residence time, loading density, and load pH.
Using a DOE approach in JMP, in combination with Pharmaron’s automated HTP robotics platform, allowed us to change all these factors at the same time. This enabled assessment of their impact on in-process attributes using an optimal number of experiments.
Ultimately, we now have rapid, low-resource decision making which accelerates movement from development into GMP faster and consequently gets patients access to life-saving medicines much more quickly.
Meg: What data analytical advancements do you see as most important for the future?
Amy: The most exciting thing for me about using data analytics in our work at Pharmaron is the power, insight, and knowledge it can give us about our products and processes. At present, these tools are mainly used within R&D, but I am eager to see them being used regularly throughout the entire life cycle of a product, from early research all the way to routine batch manufacture.
One example of where this is starting to come to fruition is through smart batch processing, where predictive analytics are combined with Process Analytical Technologies (PAT) to allow continuous monitoring of process performance, improved process understanding, and consistency in product quality. I look forward to seeing advanced data analytics become an integral part of biopharmaceutical research, development, process characterization, process validation, and even pharmacokinetic-pharmacodynamic (PK-PD) studies.
With huge developments in AI and machine learning, the mass of data we collate on our platforms and processes will soon be used to automatically adjust our operating conditions to consistently ensure our products are meeting the required quality standards. Not only is this incredibly exciting, but it will accelerate advanced therapeutics such as gene therapies into highly cost-effective, personalized medicines that are accessible to a wide range of patients, more quickly, across the globe.