Example of SEM for Summarized Data Tables
The Structural Equation Models (SEM) platform has the capability ofcan importing summarized data tables (e.g., correlation matrix) to fit a variety of models using summarized data without needing individual-level data. A summarized data table is a condensed version of raw data where the observations have been aggregated. For example, a correlation matrix is used to represent the relationships between variables rather than showing individual data points.
In this example, you will input a summarized data table, a correlation matrix published in Holzinger and Swineford (1937), into the SEM platform to fit a bi-factor model and assess the measurement model. The summarized data table includes the correlation matrix, which containsing correlations among 14 observed variables about related to cognitive ability, instead of individual test scores. The bi-factor model will fit a general cognitive ability factor (g) and multiple unique factors (spatial, mental, motor, and verbal abilities). You then use the Assess Measurement Model option to calculate Hierarchical Omega and evaluate the properties of the measurement scale's properties. The correlation matrix and sample size are sufficient forto launching the SEM platform in summarized data mode.
1. Select Help > Sample Data Folder and open Cognitive Ability I.jmp.
2. Select Analyze > Multivariate Methods > Structural Equation Models.
3. InOn the Summarized Data Format tab, select Visual Perception I through Vocabulary Test and click Model Variables.
4. In the Options panel, enter 355 in the Sample Size box.
Note: The correlation matrix and sample size are sufficient for launching the SEM platform in summarized data mode. If the summarized data table includes the Mean and Std Dev columns, then select them in the Select Columns panel and click Mean and Std Dev in the Cast Selected Columns into Roles panel.
Figure 8.16 Summarized Data Format Launch Window
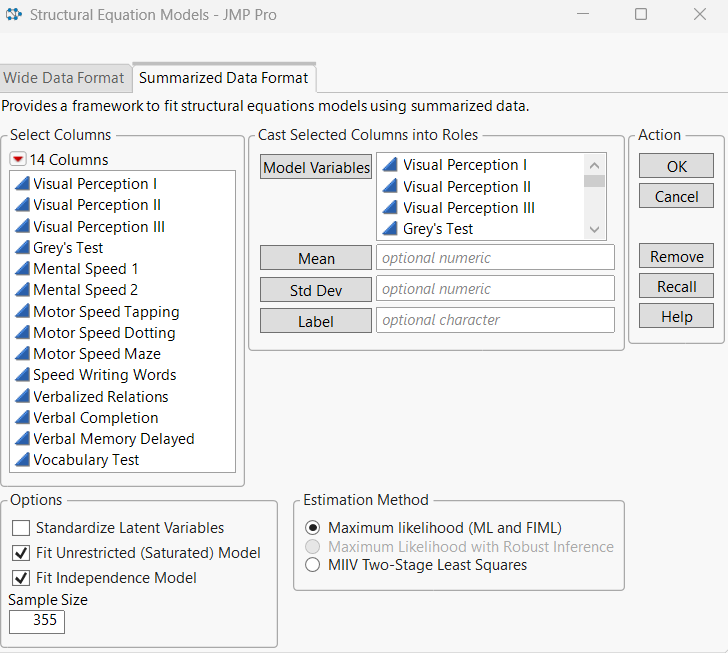
5. Click OK.
The Structural Equation Models report Model Specification outline appears.
6. Select Visual Perception I through Visual Perception III in the To List, type Spatial Ability in the box below the To List, and click the add latent variable to model
button.
7. Select Grey’s Test through Mental Speed 2 in the To List, type Mental Speed in the box below the To List, and click the add latent variable to model
button.
8. Select Motor Speed Tapping through Speed Writing Words in the To List, type Motor Speed in the box below the To List, and click the add latent variable to model
button.
9. Select Verbalized Relations through Vocabulary Test in the To List, type Verbal Ability in the box below the To List, and click the add latent variable to model
button.
10. Select Visual Perception I through Vocabulary Test in the To List, type General Cognitive Ability in the box below the To List, and click the add latent variable to model
button.
11. Click Run.
Figure 8.17 Summary of Fit for Summarized Data Format

The Structural Equation Models report that appears, includesing Summary of Fit and Parameter Estimates tables. The Summary of Fit table shows a good overall fit for the bi-factor model. The CFI value of 0.953 and RMSEA value of 0.062, both indicate that the model provides a reasonable approximation of the data. The negative BIC value suggests the model is highly parsimonious, which is a favorable indication.
Figure 8.18 Parameter Estimates for Summarized Data Format
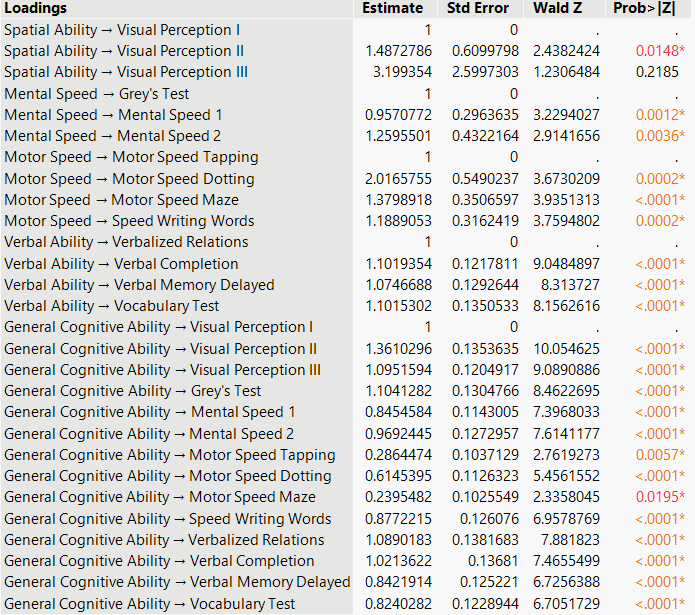
The loadings represented in the Parameter Estimates report indicate that General Cognitive Ability (g-factor) significantly influences most of the observed variables across different cognitive domains. The Uunique factors (Spatial Ability, Mental Speed, Motor Speed, and Verbal Ability) also have significant influences on their respective indicators, though the strength of these relationships varies. However, the few non-significant loadings suggest that some indicators may not be well explained by the specific factor they are intended to measure.
12. Click the red triangle next to Structural Equation Model: Model 1 and select Assess Measurement Model.
Figure 8.19 Indicator Reliability Report
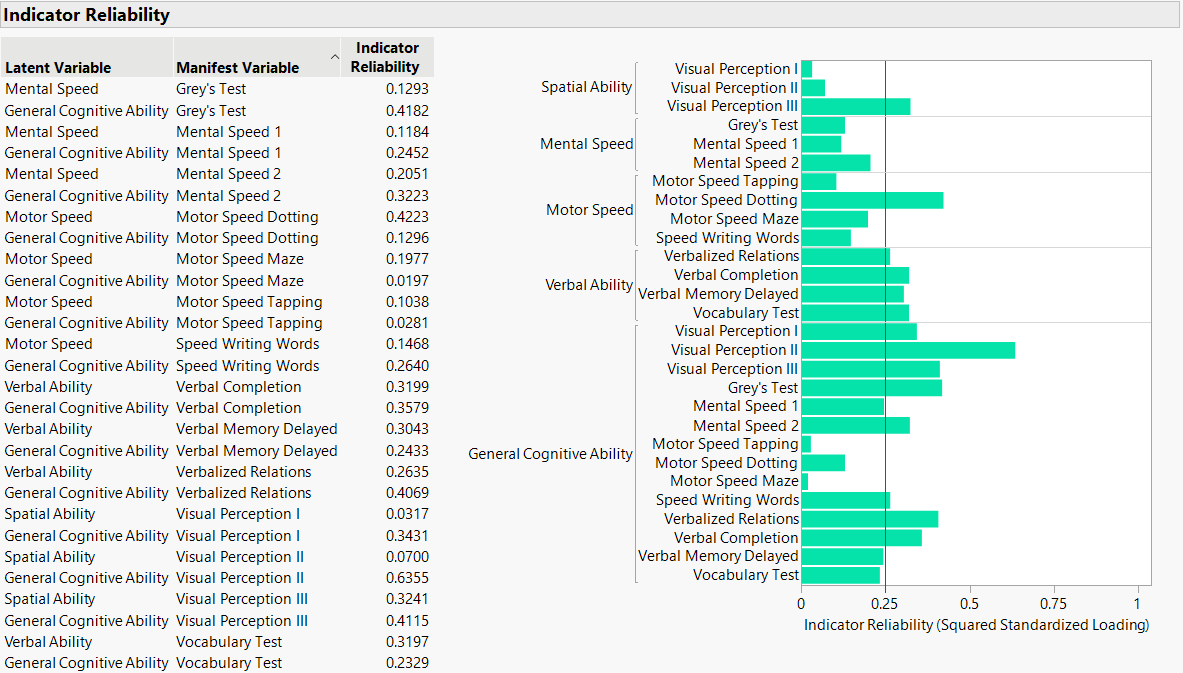
The Indicator Reliability report indicates that the General Cognitive Ability latent variable consistently explains a large portion of the variance in most of the cognitive ability tests., This suggestsing that it is a key factor that influencesing performance across different domains. Similarly, the unique latent variables like Mental Speed, Motor Speed, and Spatial Ability show varying levels of reliability, with some manifest variables (like such as Motor Speed Dotting and Verbal Completion) being better explained than others. Finally, low indicator reliability values suggest that certain latent factors may not be strong predictors for some manifest variables., This mightpossibly indicateing measurement issues or a lack of fit in those areas of the model.
The indicator reliability graph shows the squared standardized loadings for various cognitive tests, broken down by their association with both unique latent abilities (Spatial Ability, Mental Speed, Motor Speed, Verbal Ability) and General Cognitive Ability (g-factor). According to this graph, General Cognitive Ability shows stronger overall reliability across different tests compared to specific abilities. Also, tests that measureing Verbal Ability and Spatial Ability tend to have moderate to high reliability, particularly for Verbal Completion and Visual Perception II. The low reliability of some tests, particularly for Mental Speed and Motor Speed, suggests that these tests may not be the best measures for the corresponding specific abilities, but they are still captured somewhat by general cognitive ability.
Figure 8.20 Composite Reliability Report
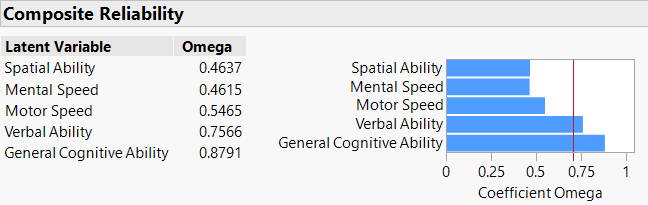
The Composite Reliability table presentscontains Omega (Hierarchical) values for different latent variables., The Hierarchical Omega values which measure the reliability of the latent constructs in the context of a bi-factor model. The Hierarchical Omega indicates how well the latent variables (unique and general) in a bi-factor model account for the variance in the observed indicators. Higher oOmega values indicate greater reliability. According to this table, General Cognitive Ability (Omega = 0.8791) is the most reliable factor in this model, and it explainsing a large portion of the variance across many indicators. Verbal Ability (Omega = 0.7566) is also highly reliable, and it showsing strong explanatory power for its corresponding verbal-related tasks. However, Motor Speed, Spatial Ability, and Mental Speed have lower omega values, which indicateing that they are less reliable in accounting for the variance in their respective indicators. These specific factors might not be as robust or may be better explained by the general cognitive ability.